Keynote Speakers
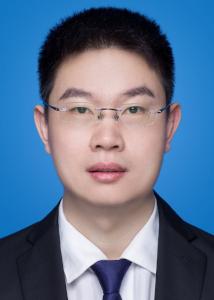
Dongrui Wu
Huazhong University of Science and Technology
Dongrui Wu received a B.E in Automatic Control from the University of Science and Technology of China, Hefei, China, in 2003, an M.Eng in Electrical and Computer Engineering from the National University of Singapore in 2005, and a PhD in Electrical Engineering from the University of Southern California, Los Angeles, CA, in 2009. He is now a Professor and Deputy Director of the Key Laboratory of the Ministry of Education for Image Processing and Intelligent Control, School of Artificial Intelligence and Automation, Huazhong University of Science and Technology, Wuhan, China. Prof. Wu's research interests include affective computing, brain-computer interface, computational intelligence, and machine learning. He has more than 140 publications (6,400+ Google Scholar citations; h=39), including a book "Perceptual Computing" (Wiley-IEEE Press, 2010), and five US patents. He received the FUZZ-IEEE Best Student Paper Award in 2005, the IEEE Computational Intelligence Society (CIS) Outstanding PhD Dissertation Award in 2012, the IEEE Transactions on Fuzzy Systems Outstanding Paper Award in 2014, the North American Fuzzy Information Processing Society (NAFIPS) Early Career Award in 2014, the IEEE Systems, Man and Cybernetics (SMC) Society Early Career Award in 2017, and the IEEE SMC Society Best Associate Editor Award in 2018. He was a finalist of the IEEE Transactions on Affective Computing Most Influential Paper Award in 2015, the IEEE Brain Initiative Best Paper Award in 2016, the 24th International Conference on Neural Information Processing Best Student Paper Award in 2017, the Hanxiang Early Career Award in 2018, and the USERN Prize in Formal Sciences in 2019. He was a selected participant of the Heidelberg Laureate Forum in 2013, the US National Academies Keck Futures Initiative (NAKFI) in 2015, and the US National Academy of Engineering German-American Frontiers of Engineering (GAFOE) in 2015. His team won the First Prize of the China Brain-Computer Interface Competition in 2019. Prof. Wu is/was an Associate Editor of the IEEE Transactions on Fuzzy Systems (2011-2018), the IEEE Transactions on Human-Machine Systems (since 2014), the IEEE Computational Intelligence Magazine (since 2017), and the IEEE Transactions on Neural Systems and Rehabilitation Engineering (since 2019).
Keynote Title
Patch Learning
Keynote Abstract
There have been different strategies to improve the performance of a machine learning model, e.g., increasing the depth, width, and/or nonlinearity of the model, and using ensemble learning to aggregate multiple base/weak learners in parallel or in series. This talk describes a novel strategy called patch learning (PL) for this problem. It consists of three steps: 1) train an initial global model using all training data; 2) identify from the initial global model the patches which contribute the most to the learning error, and then train a (local) patch model for each such patch; and, 3) update the global model using training data that do not fall into any patch. To use a PL model, one first determines if the input falls into any patch. If yes, then the corresponding patch model is used to compute the output. Otherwise, the global model is used. To-date, PL can only be implemented using fuzzy systems. How this is accomplished will be explained. Finally, some regression problems on 1D/2D/3D curve fitting, nonlinear system identification, and chaotic time-series prediction, will be explained to demonstrate the effectiveness of PL. PL opens up a promising new line of research in machine learning.
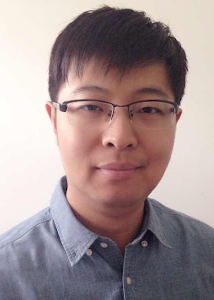
Yimin Yang
Lakehead University
Yimin Yang received the Ph.D. degree from the College of Electrical and Information Engineering, Hunan University, Changsha, China, in 2013. From 2014-2018, he was a Postdoctoral Fellow in the Department of Electrical and Computer Engineering at the University of Windsor, Ontario, Canada. He is currently an Assistant Professor at the Computer Science Department in Lakehead University, Ontario, Canada. Dr. Yang’s research interests include artificial neural networks, signal processing, and robotics. He has around 60 publications with a cumulative Impact Factor of 210, including TPAMI, TNNLS, TCYB, TII, TIM, etc. He is an Associate Editor for the IEEE Transactions on Circuits and Systems for Video Technology, and the Neurocomputing. He has been serving as a Guest Editors/Reviewers for many international journals of his research field and a Program Committee Member of some international Conferences.
Keynote Title
Artificial Neural Networks with Subnetwork Nodes: From Single-layer Network to Hierarchical Networks
Keynote Abstract
An iterative method of learning has become a paradigm for training artificial neural networks (ANN). However, utilizing a non-iterative learning strategy can accelerate the training process of the ANN. It motivates us to introduce a non-iterative learning strategy that eliminates the purpose of backpropagation in an ANN, resulting in lower training time with competitive performance. This talk will cover a neural network with subnetwork node architecture where the non-iterative learning strategy has been conducted to result in a competitive performance. The subnetwork node, which can be constituted by neurons, has been used as a fundamental unit to design artificial neural networks. Then we extend and apply this method for such application fields as dimension reduction, image processing, EEG signal recognition, etc.
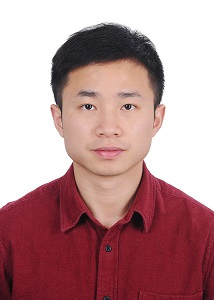
Liang Feng
Chongqing University
Liang Feng received the Ph.D degree from the School of Computer Engineering, Nanyang Technological University, Singapore, in 2014. He was a Postdoctoral Research Fellow at the Computational Intelligence Graduate Lab, Nanyang Technological University, Singapore. He is currently an Assistant Professor at the College of Computer Science, Chongqing University, China. His research interests include Computational and Artificial Intelligence, Memetic Computing, Big Data Optimization and Learning, as well as Transfer Learning. His research work on evolutionary multitasking won the 2019 IEEE Transactions on Evolutionary Computation Outstanding Paper Award. He is Associate Editor of the IEEE Computational Intelligence Magazine, Memetic Computing, and Cognitive Computation. He is also the founding Chair of the IEEE CIS Intelligent Systems Applications Technical Committee Task Force on “Transfer Learning & Transfer Optimization” and the PC member of the IEEE Task Force on “Memetic Computing”. He had co-organized and chaired the Special Session on “Memetic Computing” held at IEEE CEC’16, CEC’17, CEC’18, CEC’19, and the Special Session on "Transfer Learning in Evolutionary Computation" held at CEC’18, CEC’19.
Keynote Title
Towards Evolutionary Multi-task Optimization
Keynote Abstract
Evolutionary algorithms (EAs) typically start the search from scratch by assuming no prior knowledge about the task being solved, and their capabilities usually do not improve upon past problem-solving experiences. In contrast, humans routinely make use of the knowledge learnt and accumulated from the past to facilitate dealing with a new task, which provides an effective way to solve problems in practice as real-world problems seldom exist in isolation. Similarly, practical artificial systems like optimizers will often handle a large number of problems in their lifetime, many of which may share certain domain-specific similarities. This motivates the design of advanced optimizers which can leverage on what has been solved before to facilitate solving new tasks. In this talk, I will present recent advances in the field of evolutionary computation under the theme of evolutionary multi-task optimization via automatic knowledge transfer. Particularly, I will describe a general workflow of evolutionary multi-task optimization, which is followed by specific evolutionary multitasking algorithms for both continuous and combinatorial optimizations. Potential research directions towards advanced evolutionary multitasking design will also be covered.
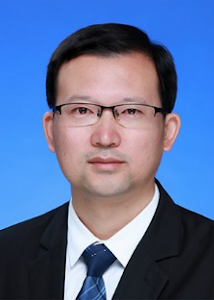
Zhong Ji
Tianjin University
Zhong Ji received the Ph.D. degree in signal and information processing from Tianjin University, Tianjin, China, in 2008. He is currently an Associate Professor with the School of Electrical and Information Engineering, Tianjin University, China. His current research interests include machine learning, computer vision, and multimedia understanding. He especially focuses on zero/few-shot learning and image-text retrieval related research topics. He has authored or co-authored more than 80 papers in refereed journals and conferences, including the IEEE TIP, IEEE TNNLS, IEEE TCYB, IEEE TCSVT, PR, NeurIPS, AAAI, IJCAI, CVPR, ICCV, and ACM MM. He has served as a program committee or area chair for many international conferences, including NeurIPS, ICML, IJCAI, ICCV, ACM MM, PCM, and NCAA. He has 25 national invention patents.
Keynote Title
Zero/Few-Shot Learning
Keynote Abstract
We live in the age of Big Data, featuring huge image and video datasets. Benefiting from this, most of the existing artificial intelligence methods achieve their successes in data-intensive applications. However, they are often hampered when the datasets are small. We call it "big data small task" data intelligence. However, the real intelligence is the intelligence that can draw inferences only from zero or few examples. Just like we humans, we are capable of learning new tasks rapidly by utilizing what we learned in the past (without any examples) or a few examples. To simulate human intelligence and realize the ability of drawing inferences and intelligent reasoning, Zero-Shot Learning (ZSL) and Few-Shot Learning (FSL) are developed and received intensive attention in recent years. Specifically, ZSL is the ability of solving a learning task without ever having been trained on it, and FSL is to learn from a limited number of examples with supervised information. This talk introduces their technical progress and shares some of our studies.
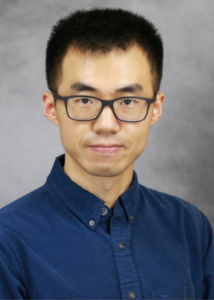
Sheng Li
University of Georgia
Dr. Sheng Li is an Assistant Professor of Computer Science at the University of Georgia since 2018. He was a Research Scientist at Adobe Research from 2017 to 2018. Prior to that, he obtained the Ph.D. degree in computer engineering from Northeastern University in 2017. Dr. Li's research interests include graph based machine learning, deep learning, user behavior modeling, visual intelligence, and causal inference. He has published over 90 papers at peer-reviewed conferences and journals, and has received over 10 research awards, such as the Aharon Katzir Young Investigator Award, Adobe Data Science Research Award, and SDM Best Paper Award. He serves as Associate Editor of five international journals including IEEE Computational Intelligence Magazine and Neurocomputing. He has also served as senior program committee member for AAAI and IJCAI, and area chair of ICPR.
Keynote Title
Machine Learning Meets Causal Inference
Keynote Abstract
Causal inference is a critical research topic across many domains, such as statistics, education, political science and economics, for decades. Estimating causal effect from observational data has become an appealing research direction owing to the large amount of available data and low budget requirement, compared with randomized controlled trials. Embraced with the rapidly developed machine learning techniques, various causal effect estimation methods for observational data have sprung up. In this talk, I will introduce how machine learning helps infer causality from observational data, and introduce classical causal inference methods as well as the recent deep learning based causal inference methods. Domain applications and future research directions will also be discussed.
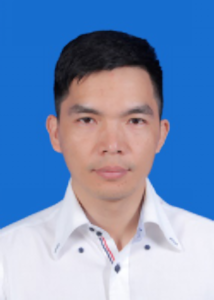
Weiwei Wu
Southeast University
Weiwei Wu received his PhD degree from City University of Hong Kong and University of Science and Technology of China in 2011. He is currently a Professor in Southeast University, P.R. China. His research interest includes game theory, reinforcement learning, multi-agent systems, wireless networks. He has published over 50 peer-reviewed papers in international conferences/journals like IJCAI, AAMAS, INFOCOM, and serves as TPCs and reviewers for several top international journals and conferences.
Keynote Title
Budget-feasible Mechanism Design
Keynote Abstract
Mechanism design is a subfield of game theory which attracts much attention in recent years. In mechanism design, one of the fundamental concerns is the incentives of participants. To introduce incentives, many efficient mechanisms are proposed in the literature where participants are usually rewarded with (possibly high) payments. However, buyers/requesters in the markets usually have budgets when they want to procure items. This falls into the field of budget-feasible mechanism design, which is initiated in recent years. In this talk, we will introduce our recent works on budget-feasible mechanism design, including both the progress in a one-sided market and two-sided markets.
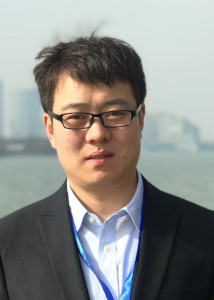
Ning Sun
Nankai University
Ning Sun received the B.S. degree in measurement & control technology and instruments from Wuhan University, Wuhan, China, in 2009, and the Ph.D. degree in control theory and control engineering from Nankai University, Tianjin, China, in 2014. He is currently an Associate Professor with the Institute of Robotics and Automatic Information Systems, College of Artificial Intelligence, Nankai University, Tianjin, China. He was awarded the prestigious Japan Society for the Promotion of Science (JSPS) Postdoctoral Fellowship for Research in Japan (Standard) in 2018. His research interests include intelligent control for mechatronic/robotic systems with emphasis on (industrial) applications. Dr. Sun received the Wu Wenjun Artificial Intelligence Excellent Youth Award in 2019, the China 10 Scientific and Technological Developments in Intelligent Manufacturing (2nd achiever) in 2019, the First Class Prize of Wu Wenjun Artificial Intelligence Natural Science Award in 2017, the First Class Prize of Tianjin Natural Science Award in 2018, the Golden Patent Award of Tianjin in 2017, the IJCAS (International Journal of Control, Automation, and Systems) Academic Activity Award twice in 2018 and 2019, the Outstanding Ph.D. Dissertation Award from the Chinese Association of Automation (CAA) in 2016, etc. He is the Executive Editor for Measurement and Control and serves as an Associate Editor (editorial board member) for several journals, including IEEE Access, Frontiers in Neurorobotics, International Journal of Control, Automation, and Systems, IET Cyber-Systems & Robotics, Transactions of the Institute of Measurement and Control, International Journal of Precision Engineering and Manufacturing, etc. Dr. Sun has been an Associate Editor of the IEEE Control Systems Society (CSS) Conference Editorial Board since July 2019, and he is an Associate Editor for IEEE/RSJ IROS 2020 and IEEE/RSJ IROS 2020 Workshops. He is presently an IEEE Senior Member and an ASME Member.
Keynote Title
Modeling, Analysis, and Control for Pneumatic Artificial Muscle Robots
Keynote Abstract
With the rapid development of rehabilitation robots and the growing demands for human-robot interaction, modeling and intelligent control of pneumatic artificial muscle (PAM) robots have increasingly attracted the attention of many researchers. It is a challenging research topic to overcome the effects of PAMs’ inherent defects (e.g., high nonlinearities, hysteresis, time-varying characteristics, etc.), despite the merits of lightness, safety, and high power-to-weight/volume ratios of PAMs. To this end, we aim to achieve accurate modeling and advanced control for PAM robots, which may contribute to their further theoretical research and practical applications. Specifically, for single-PAM robots, there exist some difficulties as follows: 1) PAM systems are susceptible to unknown external disturbances due to their high nonlinearities, creep, hysteresis, etc. 2) PAM robots usually suffer from parameter uncertainties and unmodeled dynamics. 3) The ultimate control inputs (corresponding to the pressurized air) of PAM robots should be constrained to be nonnegative. To solve these problems, we propose a disturbance estimation-based nonlinear control method, a neuroadaptive control method with system uncertainties, and an adaptive control method with unidirectional input constraints, respectively. Further, for multi-PAM robots, the following issues should be considered: 1) Since torques/forces are generated by air pressure and are not the ultimate control inputs, the torque models of PAM robots are not direct and effective. 2) To ensure safety, the system state variables (e.g., contracted lengths of muscles, ranges of robots’ movements, etc.) are usually limited. To this end, we propose an accurate dynamic modeling method and a nonlinear control method with overshoot constraints, respectively. Some future research directions will also be discussed.
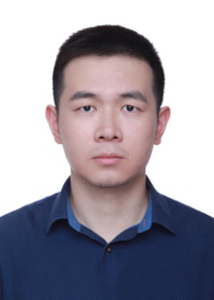
Xi Peng
Sichuan University
Xi Peng is currently a Professor of Sichuan University. He has served as an Associate Editor (AE) for three peer-reviewed journals including IEEE Trans on SMC: Systems. He is the Deputy Secretary-General of the Youth Working Committee of the China Society of Image and Graphics (CSIG), the Secretary-General of the Member Services and Development Working Committee, and the Program Chair of Vision and Learning Seminar (VALSE). His current research interests include machine learning and its applications in multimedia analysis, computer vision, and natural language processing, and in these areas he has co-/authored over 50 papers.
Keynote Title
Multi-modal Representation Learning
Keynote Abstract
Multi-modal or cross-modal representation learning aims to learn the intrinsic representation of multi-modal data by exploiting the complementary and consistent information of different modalities. On the learned data representation, a series of applications, such as classification, clustering, retrieval, 3D modeling, action identification, and object re-identification could be conducted. In this talk, I will introduce two recent works of my group on multi-modal representation learning. The first work shows a feasible solution to learning multi-modal data representation in a neural network. Specifically, we reformulate the orthogonal constraint as a novel differentiable layer such that the vanilla spectral clustering could be reformulated into a neural network. In another work, we propose utilizing the consistency existing in cross-modal data to solve the model selection problem. To be specific, the proposed method could adaptively learn all parameters including the number of clusters for multi-modal clustering. The work could be one of first attempts to solve the model selection problem in multi-modal clustering, which has achieved state-of-the-art performance in extensive evaluations.