Keynote Speakers
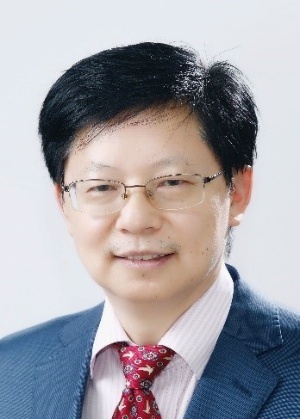
Yongduan Song
Chongqing University
Yongduan Song is an IEEE Fellow and a Registered Professional Engineer (USA). He has published 12 monographs and 300 papers. He also holds more than 80 patents. He served/has been serving as Associate Editor for several internationally renowned academic journals, including IEEE Trans. On Automatic Control, IEEE Trans. on Intelligent Transportation Systems, etc. He is the Editor-in-Chief of IEEE Transactions on Neural Networks and Learning Systems. His current research interests include advanced control theory, artificial intelligence and the related applications.
Keynote Title
Neuroadaptive PID Control with Application to Unmanned Systems
Keynote Abstract
Proportional-Integral-Derivative (PID) Control has been widely utilized in many engineering systems due to its simplicity in structure and intuition in concept. However, its applicability to higher order uncertain nonlinear systems remains unclear. This talk is focused on PID control of nonlinear MIMO systems under actuation faults and modeling uncertainties. Neuroadaptive algorithms for PID gain tuning will be presented and discussed. Application to unmanned systems will be demonstrated.
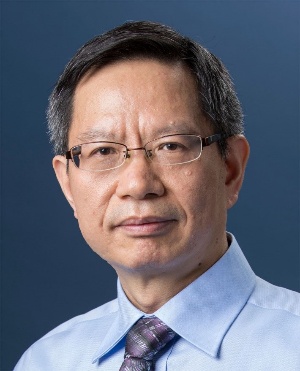
Cheng-Lin Liu
Institute of Automation of Chinese Academy of Sciences
Cheng-Lin Liu is a Professor at the National Laboratory of Pattern Recognition, Institute of Automation of Chinese Academy of Sciences, and now the Director of the Laboratory. He is a vice president of the Institute of Automation, a vice dean of the School of Artificial Intelligence, University of Chinese Academy of Sciences. He received the PhD degree in pattern recognition and intelligent control from the Chinese Academy of Sciences, Beijing, China, in 1995. He was a postdoctoral fellow in Korea and Japan from March 1996 to March 1999. From 1999 to 2004, he was a researcher at the Central Research Laboratory, Hitachi, Ltd., Tokyo, Japan. His research interests include pattern recognition, machine learning and document image analysis. He has published over 300 technical papers at journals and conferences. He is an Associate Editor-in-Chief of Pattern Recognition Journal and Acta Automatica Sinica, an Associate Editor of International Journal on Document Analysis and Recognition, Cognitive Computation, IEEE/CAA Journal of Automatica Sinica, CAAI Trans. Intelligence Technology, CAAI Trans. Artificial Intelligence and Chinese Journal of Image and Graphics. He is a Fellow of the CAA, CAAI, the IAPR and the IEEE.
Keynote Title
Deep Prototype Learning for Classification and Generalization in Open World
Keynote Abstract
Traditional classification and machine learning assumed that the input patter belongs to a set of pre-defined classes. This does not hold in open world, where the input many be novel (out of know classes), and the class set may change. The related problems like open set recognition, class incremental learning, few-shot and zero-shot learning, have been studied using deep learning methods. We proposed a deep network called convolutional prototype network (CPN) for performing these tasks. The CPN is shown to learn a feature space making samples between-class separable and within-class compact, so that novel patterns can be rejected according to nearest distance. By learning new-class prototypes while memorizing old-class prototypes, the CPN can yield superior performance in class-incremental learning, few-shot and zero-shot learning.
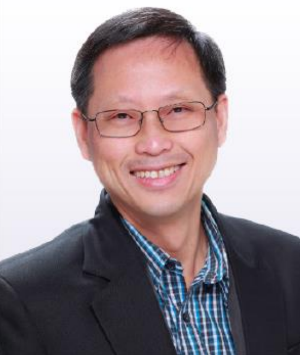
Kim-Fung Tsang
City University of Hong Kong
Dr Tsang is an Associate Professor in the Department of Electronic Engineering, City University of Hong Kong. He has published more than 250 technical papers, four books/book chapter and some patents. KF's research area is in RF circuit and system design, wireless protocol design, AI algorithms, IoT system and applications. KF was awarded the Internet of Things Heros Award in 2016. KF is the Editor-in-Chief of Transactions on Consumer Electronics; an Associate Editor of IEEE Transactions on Industrial Electronics; Associate Editor of IEEE Transactions on Industrial informatics; Associate Editor of IEEE Industrial Electronics Magazine; Editor (Electrical) of the HKIE Transactions; Editor of KSII Transactions on Internet and Information Systems, Editor of SmartVision, Smart City Consortium. He is also the President of Greater Bay Area 5G Industry Alliance, Honorary Advisor Committee; Honorary President of the Connected Cities Alliance; Past Chairman of HKIE Electronics Division, Council Rep (Electronics Div), HKIE; Ad Hoc Technical Committee, Smart Lamppost, OGCIO; member SSAC, OFCA.
Keynote Title
Internet of Things Maturity Index Elevating Digital Economy
Keynote Abstract
Thanks to the tremendous progress of the Internet of Things (IoT) and the IoT-related technologies, the global market has been shifting towards high-connectivity and high-intelligence applications. It is anticipated that the number of IoT devices would exceed 50 billion by 2025, forming a trillion-dollar IoT market. The IoT has established a high-level framework for the integration of physical and cyber objects for seamless information exchange and ubiquitous data access. Numerous smart applications and infrastructure have been driven, to name a few, smart healthcare, smart grids, smart buildings, smart cities, etc. There will be plentiful IoT connections and use cases, and challenges would be encountered. Some problems are yet to be solved, such as the IoT standardization, the performance evaluation of IoT products, the IoT security and safety, etc. The Internet of Things Maturity Index, referred to as the IDex, has been developing to cater for all these needs. The IDex, defined by the IEEE Standards Working Group P2668, aims to measure the maturity of IoT objects, covering devices, networks, applications, services, etc., in a quantitative, objective, trustworthy, and standardized manner. The IDex would classify and quantify the maturity of an IoT object into multiple levels with respect to the object’s specification and application. In addition, the IDex would standardize a universal IoT architecture to achieve high interoperability between various IoT objects, thus minimizing the development effort on solving the compatibility issues and proliferating digital integration. Reliability is another essential feature to sustain the growth of the digital economy. Risk analysis would take a critical role in which the IDex could help quantify the IoT risk levels. For instance, smart applications and infrastructure would exploit a huge amount of data for analysis and decision-making. Availability is one of the critical parameters to evaluate the reliability of smart applications and infrastructure. Distributed Denial of Service (DDoS) attack is a common cyber-attack that would significantly influence availability. The IDex quantifies the severity of DDoS attacks to facilitate operators to perform necessary security measures. Recent research on IDex methodology will be discussed. Examples of IDex applications and show cases will also be given.
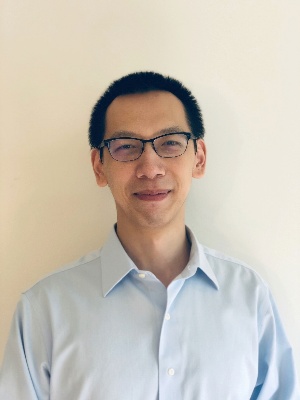
Xiaowei Jia
University of Pittsburgh
Xiaowei Jia is an Assistant Professor in the Department of Computer Science at the University of Pittsburgh. He obtained his Ph.D. degree at the University of Minnesota, under the supervision of Prof. Vipin Kumar. Prior to that, he received his M.S. degree from State University of New York at Buffalo and his B.S. degree from University of Science and Technology of China. His research interests include spatio-temporal data mining, physics-guided data science, and deep learning. He has published over 70 papers in major journals in data mining (e.g., TKDE) and scientific journals, as well as top-tier conferences (e.g., SIGKDD, ICDM, SDM, and CIKM). Prof. Jia was the recipient of the University of Minnesota Best Dissertation Award, the Best Application Paper Award in SDM 2021 and 2022, the Best Conference Paper Award in ICDM 2021 and ASONAM 2016, and the Best Student Paper Award in BIBE 2014.
Keynote Title
Physics Guided Machine Learning for Scientific Knowledge Discovery
Keynote Abstract
Data science and machine learning (ML) models, which have found tremendous success in several commercial applications where large-scale data is available, e.g., computer vision and natural language processing, has met with limited success in scientific domains. Traditionally, physics-based models of dynamical systems are often used to study engineering and environmental systems. Despite their extensive use, these models have several well-known limitations due to incomplete or inaccurate representations of the physical processes being modeled. Given rapid data growth due to advances in sensor technologies, there is a tremendous opportunity to systematically advance modeling in these domains by using machine learning methods. However, capturing this opportunity is contingent on a paradigm shift in data-intensive scientific discovery since the “black box” use of ML often leads to serious false discoveries in scientific applications. Because the hypothesis space of scientific applications is often complex and exponentially large, an uninformed data-driven search can easily select a highly complex model that is neither generalizable nor physically interpretable, resulting in the discovery of spurious relationships, predictors, and patterns. This problem becomes worse when there is a scarcity of labeled samples, which is quite common in science and engineering domains. My work aims to build the foundations of physics-guided machine learning by exploring several ways of bringing scientific knowledge and machine learning models together. In particular, we show the effectiveness of the proposed methods in predicting water temperature in lake and river systems. My work also has the potential to greatly advance the pace of discovery in a number of other scientific and engineering disciplines where physics-based models are used, e.g., hydrology, agriculture, climate science, materials science, power engineering and biomedicine.
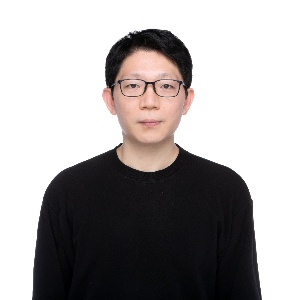
Tao Song
China University of Petroleum
Tao Song is a full Professor at China University of Petroleum, and also elected as EU-Spain Juan de la Cierva supported senior researcher in Polytechnical University de Madrid. Now he is the Deputy Dean of School of Computer Science and Technology and Qingdao Software Institute.
Keynote Title
When Artificial Intelligence Meets Drug Discovery
Keynote Abstract
Drug discovery and development can be described as the sum total of steps taken by research-intensive entity to identify a new chemical or biological substance and transform it into a product approved for use by patients. Artificial Intelligence, known as the most powerful technical tool, can speed up the process of drug discovery, such as molecule generation, drug-target Interaction prediction, drug-drug interaction prediction, protein-protein interaction prediction, and knowledge graph in drug-target. And it would be a scientific way for empowering traditional Chinese medicine going with modern medical manners.