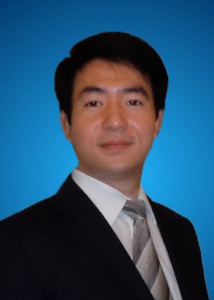
Tianyong Hao
South China Normal University
Dr. Tianyong Hao is a full Professor at South China Normal University. He received his Ph.D. degree at City University of Hong Kong in 2010. He studied and worked at York University, Emory University, University of New South Wales, and Columbia University until 2014. Dr. Hao is a senior member of IEEE/CCF and committee member of ISO TC37, SAC TC52 (associate secretary), CCF NLP, CIPS Health Informatics (associate secretary), etc. He is the lead guest editor of several SCI journals including JMIR Medical Informatics and also serves for IEEE TII, ACM TIST, KAIS, as well as conferences such as AAAI, IJCAI, ACL, COLING, etc. He has published more than 100 SCI/EI indexed papers including IEEE TKDE, ACM TALIP, JBI, KBS, C&E, etc. He is the PI of 2 grants from National Science Foundation of China and the leader of provincial level research team on NLP for big data. He owns 7 best paper awards and 2 international standards.
Keynote Title
Large Scale Clinical Trial Text Processing and Mining
Keynote Abstract
Clinical trials generate highly relevant evidences for effective disease treatments. The extraction of necessary information from a large scale clinical trial text through natural language processing for patient characteristic aggregation remains a research problem due to the complex of the investigator-authored free-text. By collaborating with a research group at Columbia University Medical Center, our research group has made some progresses on several research topics. This talk will introduce the recent research on: an extensible approach for automated semantic tag mining, clinical trial clustering by similar eligibility criteria, disease named entity recognition, temporal expression extraction and normalization, transgender identification for enhancing clinical trial recruitment, and measurable quantitative information extraction and normalization.
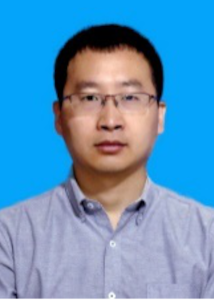
Zhao Zhang
Hefei University of Technology
Zhao Zhang is a Huangshan Scholar Distinguished Professor at the School of Computer Science and Information Engineering, Hefei University of Technology (HFUT), Hefei, China. He received the PhD degree from City University of Hong Kong in 2013. He visited the National University of Singapore and National Laboratory of Pattern Recognition (NLPR) of Chinese Academy of Sciences (CAS) in 2012. His current research interests include Machine Learning & Data Mining, Pattern Recognition & Image Processing. He has authored/co-authored more than 90 technical papers published at prestigious journals and conferences, including 32 IEEE/ACM Transactions regular papers. Dr. Zhang is serving as an Associate Editor (AE) of IEEE Transactions on Image Processing and Elsevier Signal Processing, and is serving/served as a Managing Guest Editor (MGE) for Journal of Visual Communication and Image Representation, Image and Vision Computing. Besides, he has been acting as a Senior PC member/Area Chair of IJCAI、SDM、ECAI、BMVC and PAKDD, and a PC member for 10+ popular prestigious conferences (e.g., KDD、ICDM、ACM MM、IJCAI、AAAI、CVPR、ICCV、ECCV and SDM). He is now a Senior Member of the IEEE and CCF. Personal homepage: https://sites.google.com/site/cszzhang
Keynote Title
Robust Low-Rank Representation Learning: From Shallow to Deep Models
Keynote Abstract
Representation learning mainly explores the technologies that can automatically discover potential compact expressions and explanatory factors from original complex visual data for feature detection or classification. Because the visual data in real world generally have the issues of high dimensionality, complicated content information, redundant information and unfavorable features, it is still an important and challenging research topic to effectively represent, understand and classify them. The presenter mainly focuses on the study of representation learning and its application in visual data analysis and image processing, which mainly discusses how to extract or capture useful feature subspaces from complex visual data to achieve efficient representation and understanding. In this talk, the speaker will introduce their recent progresses on robust representation learning by low-rank coding (from shallow to deep models), including some applications to image processing and pattern recognition.